Blog
The future of market research: navigating the AI-paved path ahead
Annelies Verhaeghe, our Chief Platform Officer shares her thinking on the future of AI, talking about synthetic data, insight activation and training models on owned data.
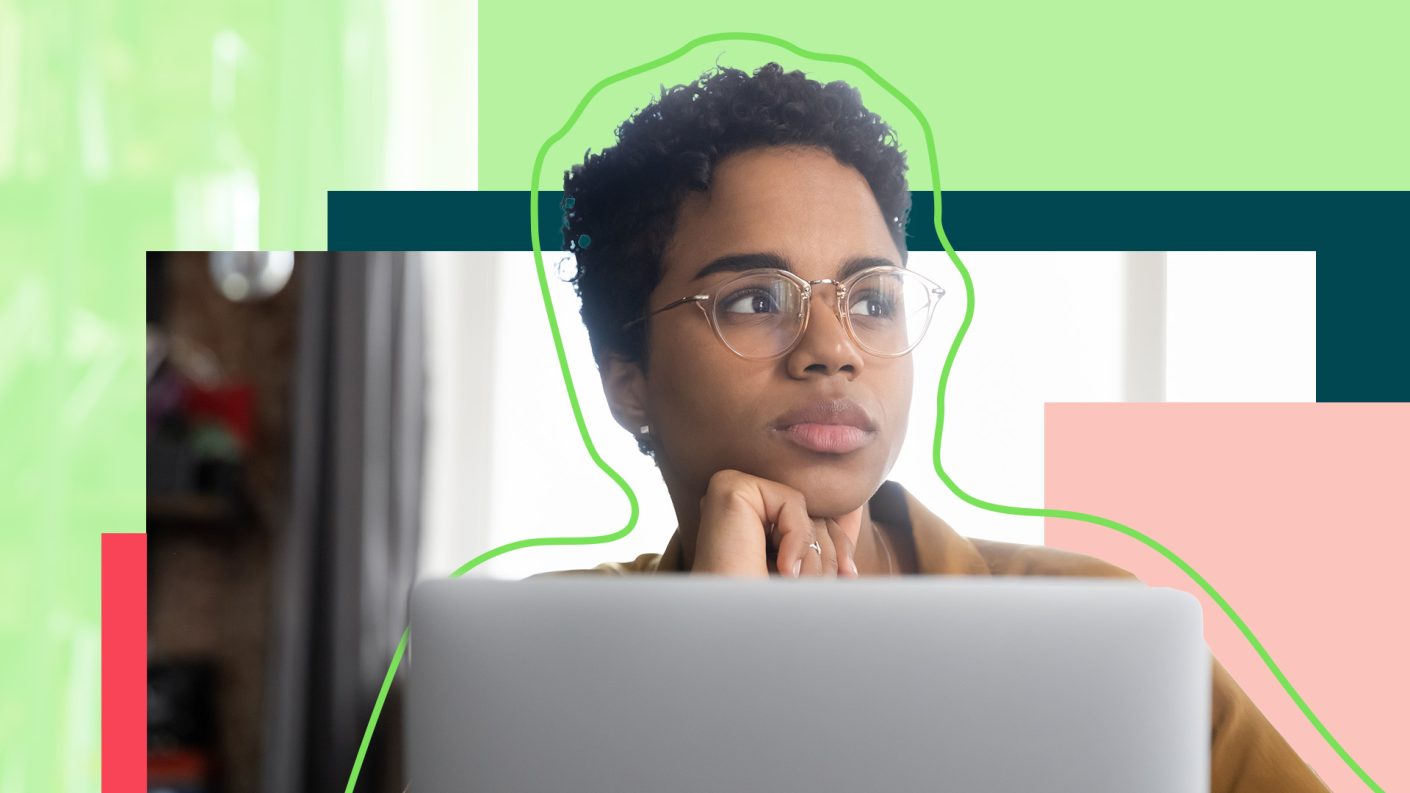
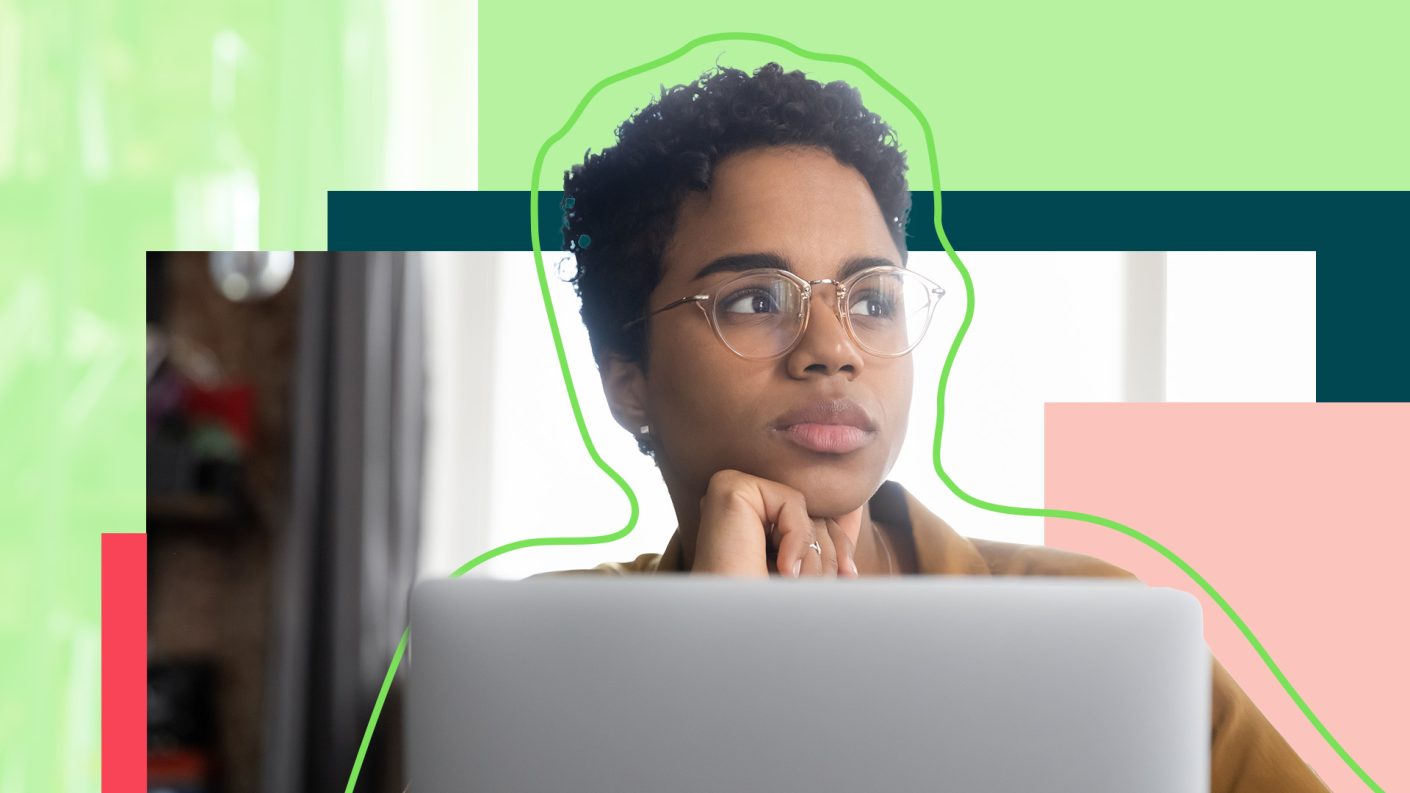
Sarah Van Oerle
23 August 2023
5 min read
We cannot ignore the recent buzz surrounding generative AI in market research. From supporting desk research to generating survey questions, moderating insight communities and analysing complex data – the use cases are endless and embraced by our industry with great enthusiasm. But caution is needed as artificial intelligence in the wrong (untrained) hands will only result in artificial stupidity.
In this blogpost, we interview Annelies Verhaeghe, Managing Partner and Chief Platform Officer at Human8. She shares her vision on the future of AI in the market research industry, touching upon the value of synthetic data, how AI can boost insight activation and what the value is of training AI models on proprietary data.
The perfect blend: complementing synthetic data with human data
Hello Annelies, before we dive right in, can you briefly explain what synthetic data is?
“Synthetic data is artificially generated through machine learning techniques, rather than being observed and collected from real-world sources. To create marketing persona, for example, researchers train AI models using extensive internet data from publicly available sources, such as purchase data, but also national statistical and demographic data. The idea is that people’s personality traits and interests can predict their behaviour. But gathering this data in the real world through traditional methods requires time and budget. This is where synthetic persona, or synthetic data in general, come into play.
Advancements in generative AI tools make generating synthetic data more accessible. But the quality of such data depends on the quality of the underlying model and datasets. Additional verification steps are necessary to ensure reliability and validity.”
In the market research industry, this might raise the question: is primary research still necessary? Why not rely solely on generative AI to predict the right price for a product, understand typical usage patterns, or identify category entry points?
“The answer is straightforward: Yes, primary research remains essential. There are multiple reasons. First, generative AI tools might not work with the latest data. Think for example about ChatGPT that relies on data up until late 2021. Second, systems cannot be prompted for things we are unaware of. To unveil the unknown, primary research is crucial. Third, AI’s sources for generating output are unclear, raising questions about data ownership and whose opinions are represented. Finally, AI acts purely rational and cannot predict irrational, emotional responses or detect say-do gaps.
In-the-moment and emotional primary data is thus vital to complement synthetic data and guide business decisions. AI models are as smart as the quality of the input they receive. Since many will use algorithms trained on the same data, high-quality primary data will become the true differentiator.
We did a test in a community around the cost-of-living crisis asking what the first things were people were cutting out of their everyday life. While AI predicted people would save on non-essentials such as travel and dining out, our primary data showed a different picture. The community members described how they were saving on essentials such as heating and groceries to still be able to enjoy the small luxuries in life. Since synthetic data relies on pure rational models, it cannot identify potential say-do gaps that exist around certain sensitive topics such as the cost-of-living crisis. People might say one thing and do another. Humans are not always rational.
Other researchers set up an A/B test to compare synthetic data with real human responses in a survey – asking ChatGPT to respond on a Likert-scale and generate verbatim responses. Also here, the conclusion was that the power lies in blending AI power with human power to supercharge research since synthetic data lacks nuance and comes with many biases.”
Democratising insights or boosting insight activation?
There is a lot of talk in the industry about how AI tools will democratize insights. What is your take on that?
“Indeed, many insight professionals are convinced that AI will increase the level of DIY and commoditization in our industry. I have two major concerns with this idea. First, we need to be aware that people need to be trained to use AI tools in a smart way. Without a thorough understanding of the context, it’s easy to draw wrong conclusions. And second, as I said before, everyone uses tools that are based on the same underlying algorithms. This will lead to similar output. If insight professionals all build further on the same insights, this will erode the potential competitive advantage of consumer insights.
I believe the greater value lies in using AI models as activation tools, bringing large groups of internal stakeholders closer to the people who are important for your brand. Just think about how the models can be trained on your own primary data to create personas that are tailored to your brand and category. Instead of reading a long report, stakeholders can quickly chat with one of the personas to immerse in their world and get answers to their most burning questions.”
The secret weapon: training AI models on owned data
You talked about the value of primary data as competitive advantage – to complement synthetic data, or to train AI chatbots for insight activation. How do you see this play out in the future?
“Training AI models on owned, primary data will be the secret weapon of the future. These models can be fine-tuned to address the specific challenges and opportunities of your organization, leading to much more accurate and tailored insights. We are also experimenting with training our personal AI research assistant on insight community data for different use cases. Think about identifying main themes in conversations, unearthing insights, but also outlier detection. Evidently, the content will not be shared across projects, nor will insights be shared across clients. It’s within a project that we aim to train our models to get the most out of community data.
But training these models also entails a significant responsibility to make ethical decisions. If not managed carefully, the systems can perpetuate societal prejudices. So having diverse and representative datasets, along with continuous monitoring, is key to mitigate potential biases. It’s important to be able to explain all the decisions you make and to be transparent about them. For instance, you could deliberately opt to give more weight to the voices of minority groups when training your AI model. If you have valid reasons for doing so and you disclose this information, it can be a sound decision for your business. Transparency is paramount.”
AI is rightfully generating a lot of excitement in the research industry. According to Annelies the way forward is about blending the power of AI with the power of human data and skills to arrive at sharp insights.
Explore more about AI in our blogs covering the AI revolution, how AI supercharges research and tips on crafting effective AI prompts.
Ready to do what matters?
Let’s connect